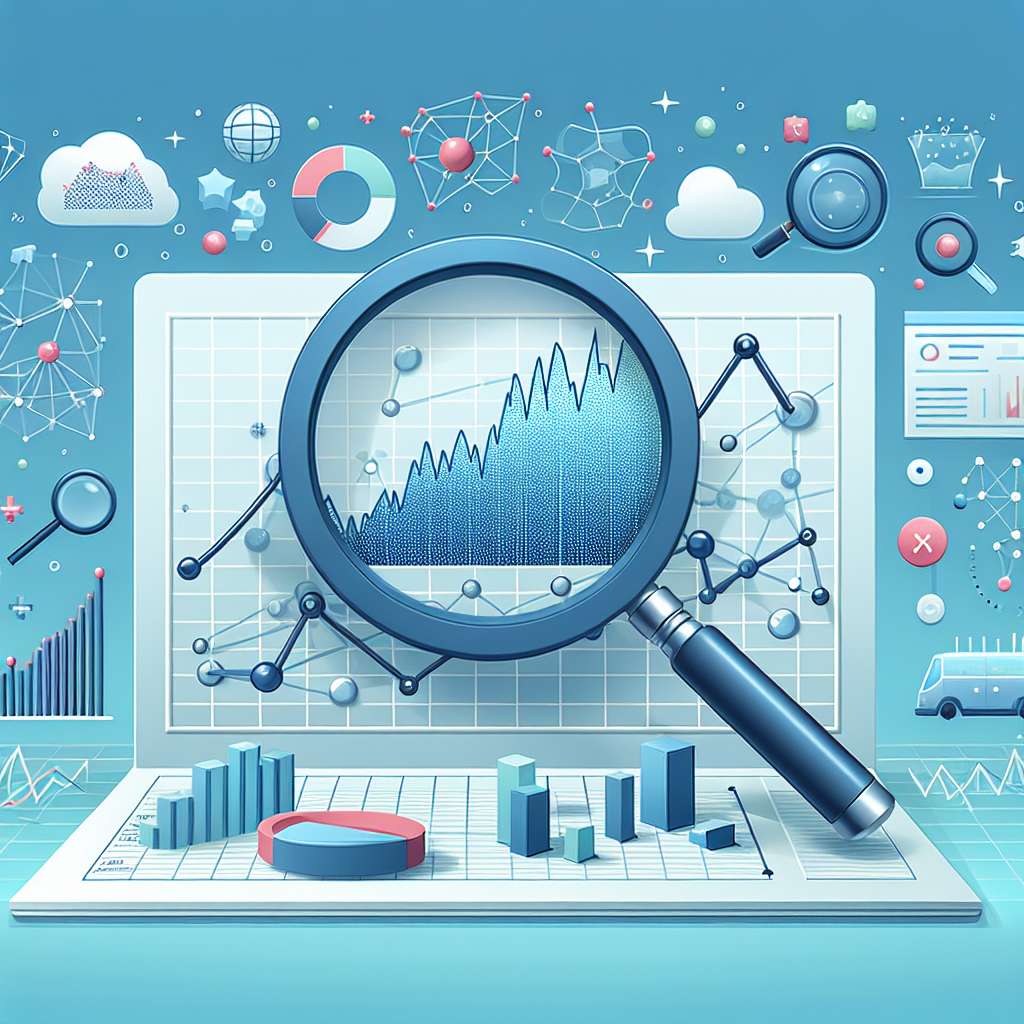
Introduction
Imagine you’re scrolling through your favorite social media feed, and you come across a post that claims, “Eating chocolate leads to better academic performance!” At first glance, the assertion may seem enticing, sparking a rush of chocolate enthusiasts to dream of A+ grades. However, this scenario raises an important question: does the mere correlation between chocolate consumption and high grades imply a causal relationship? This concept, navigating the intricate landscape from correlation to causation, forms the crux of our discussion today.
Understanding the role of observational studies is essential in deciphering the complexities of this relationship. In this article, we will unravel the nuances associated with observing correlations, analyze notable case studies, and clarify how observational studies contribute to our understanding of causality. By the end, you’ll grasp not just the science behind it but its practical implications in real-world scenarios.
The Basics: Correlation vs. Causation
What Is Correlation?
Correlation is a statistical measure that indicates the extent to which two or more variables fluctuate together. It is quantified using a correlation coefficient, which ranges from -1 to +1. A positive correlation (closer to +1) suggests that as one variable increases, the other does as well, while a negative correlation (closer to -1) indicates that as one variable increases, the other decreases.
Table 1: Correlation Coefficients
Correlation Coefficient | Description |
---|---|
+1 | Perfect positive correlation |
0 | No correlation |
-1 | Perfect negative correlation |
What Is Causation?
Causation, or causal relationship, implies that one event is the result of another; in essence, A causes B. Establishing causation is substantially more complex than recognizing correlation due to potential confounding variables.
The Perils of Misinterpretation
Misinterpreting correlation as causation is a common pitfall. The phrase “correlation does not imply causation” serves as a crucial reminder in both scientific research and everyday reasoning.
The Role of Observational Studies
Observational studies are a cornerstone in research that facilitate the examination of relationships between variables in their natural settings without manipulation. They can provide valuable insights, especially in fields like medicine, sociology, and economics.
Types of Observational Studies
-
Cohort Studies
- Follow a group over time to observe how different exposures affect outcomes.
-
Case-Control Studies
- Compare subjects with a specific condition to those without, analyzing past exposure to potential risk factors.
- Cross-Sectional Studies
- Analyze data from a population at a specific point in time, offering a snapshot of relationships.
Why Observational Studies Matter
Observational studies excel in contexts where controlled experiments are unethical or impractical. For example, conducting a randomized controlled trial on smoking and lung cancer would be unethical; thus, researchers rely on observational studies to gather data.
Case Study: Smoking and Lung Cancer
The link between smoking and lung cancer is famously established through observational studies. Researchers observed higher lung cancer rates among smokers compared to non-smokers, identifying a strong correlation. However, to claim causation, further investigation into confounding factors, such as genetic predispositions, was essential.
Analyzing Real-World Applications
Observational studies can reveal patterns that lead to fruitful inquiries. Let’s explore some case studies where these studies significantly contributed to scientific knowledge.
Case Study 1: The Framingham Heart Study
The Framingham Heart Study has provided invaluable insights into cardiovascular risks since its inception in 1948. By observing a cohort of participants over decades, researchers identified key factors such as high blood pressure, sedentary lifestyles, and smoking as contributors to heart disease.
Relevance: This long-term observational study helped transition from correlation to understanding causation regarding heart disease and lifestyle factors.
Risk Factor | Strength of Correlation with Heart Disease |
---|---|
High Blood Pressure | Strong |
Smoking | Strong |
Elevated Cholesterol | Moderate |
The Importance of Robustness
The robustness of observational studies is crucial. The more studies consistently find similar correlations, the higher the likelihood others will accept them as evidence of causation. Rigorous methodology and comprehensive statistical analysis enhance the credibility of findings.
Navigating Confounding Variables
Confounding variables complicate the correlation-causation debate. Understanding their role is essential for accurate interpretations.
What Are Confounding Variables?
A confounding variable is an external influence that skews the relationship between two observed variables. For instance, when studying the link between exercise and weight loss, dietary habits may be a confounding factor. Observational studies must account for these variables to clarify true relationships.
Strategies to Minimize Confounding
Researchers often employ several strategies to address confounding variables in observational studies:
-
Stratification
- Segregating data based on potential confounding factors for separate analysis.
- Multivariable Regression Models
- Adjusting for various confounding variables in the analysis phase.
Case Study: The Role of Education in Health Outcomes
A study examining the relationship between education level and health outcomes initially identified a strong correlation. However, subsequent analysis revealed confounding variables, including income and access to healthcare. By employing stratification, researchers found that education directly affected health outcomes, independent of other influences.
Transitioning from Observational to Causal Inference
While observational studies lay the groundwork for understanding relationships, inferring causation requires additional rigor.
The Bradford Hill Criteria
The Bradford Hill criteria are pivotal in assessing causal relationships. These nine criteria provide a framework for determining whether a correlation is strong enough to suggest causation.
- Strength: A strong association increases the likelihood of causation.
- Consistency: Repeated observations across different populations bolster causation claims.
- Specificity: A cause leads to a specific effect.
- Temporality: The cause precedes the effect.
- Biological Gradient: A dose-response relationship is observed.
- Plausibility: The causal relationship is biologically plausible.
- Coherence: The relationship does not conflict with existing knowledge.
- Experiment: Evidence from experiments supports the causal inference.
- Analogy: Similar relationships are observed in related phenomena.
A Practical Application of the Criteria
One classic example is the relationship between sunlight exposure and vitamin D levels. Multiple studies consistently link increased sunlight exposure to higher vitamin D levels, following the Bradford Hill criteria and suggesting a causal relationship.
Limitations of Observational Studies
While observational studies are invaluable, they are not without their limitations.
Potential Biases
- Selection Bias: If the sample is not representative of the general population, it can skew results.
- Observer Bias: Personal biases of researchers can influence observations and interpretations.
- Recall Bias: Participants may inaccurately report past behaviors affecting study results.
The Importance of Triangulation
Triangulation, employing multiple methodologies to examine a phenomenon, can mitigate these biases. Combining observational studies with randomized controlled trials or qualitative interviews enriches the data landscape.
Conclusion
Navigating the complex landscape from correlation to causation is critical in research and everyday decision-making. Observational studies serve as valuable tools that help us uncover correlations and begin to understand underlying causal relationships. Significant findings, such as the link between smoking and lung cancer or the Framingham Heart Study’s insights into heart disease, showcase the power of rigorous observational research.
As you engage with data and assertively question claims, remember the importance of distinguishing correlation from causation. Knowledge is power, and understanding how observational studies contribute to this journey equips you with insights to make informed decisions.
FAQs
1. What is the primary difference between correlation and causation?
Correlation indicates a statistical relationship between two variables, while causation implies that one variable directly affects another.
2. Can observational studies prove causation?
Observational studies can suggest causation, but they cannot definitively prove it due to potential confounding variables and biases.
3. How are confounding variables managed in observational studies?
Researchers use techniques like stratification and multivariable regression to account for confounding variables in their analyses.
4. What are the Bradford Hill criteria?
The Bradford Hill criteria are a set of nine principles used to assess whether an association is likely to be causal.
5. Are observational studies reliable?
While they can provide valuable insights, observational studies must be carefully designed and interpreted to minimize biases.
As we conclude this exploration into the intricate relationship between correlation and causation, we hope that you feel better equipped to interpret research findings in various domains of life.